
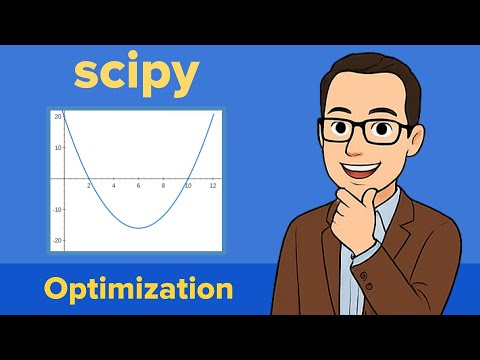
Least-squares minimization (leastsq()) and curve fitting (curve_fit()) algorithms Global (brute-force) optimization routines (e.g., anneal(), basinhopping()) BFGS, Nelder-Mead simplex, Newton Conjugate Gradient, COBYLA or SLSQP) Unconstrained and constrained minimization of multivariate scalar functions (minimize()) using a variety of algorithms (e.g. This module contains the following aspects −
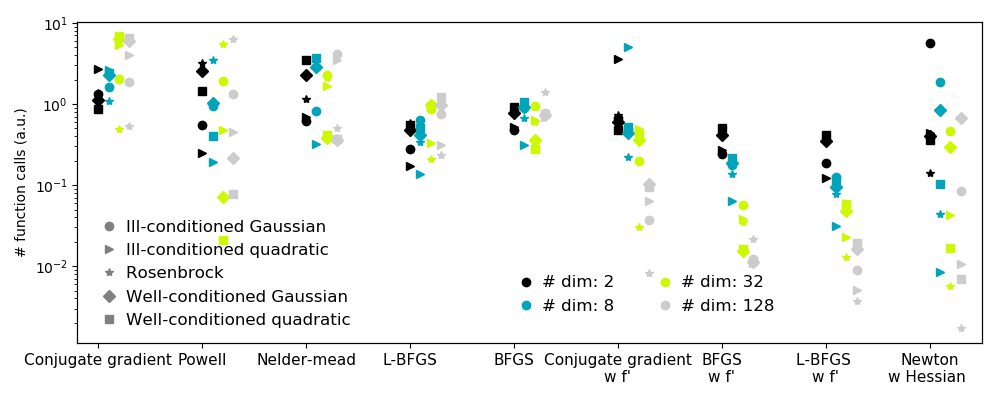
Knoll and D.E.The scipy.optimize package provides several commonly used optimization algorithms. Some further reading and related software: ĭ.A. Lot more depth to this topic than is shown here. In making a simple choice that worked reasonably well, but there is a Preconditioning is an art, science, and industry. it can even decide whether the problem is solvable in practice or Residual is expensive to compute, good preconditioning can be crucial Using a preconditioner reduced the number of evaluations of the

x def main (): sol = solve ( preconditioning = True ) # visualize import matplotlib.pyplot as plt x, y = mgrid plt. max () print 'Evaluations', count return sol. mean () ** 2 # solve guess = zeros (( nx, ny ), float ) sol = root ( residual, guess, method = 'krylov', options = ) print 'Residual', abs ( residual ( sol. ( ny - 1 ) P_left, P_right = 0, 0 P_top, P_bottom = 1, 0 def residual ( P ): d2x = zeros_like ( P ) d2y = zeros_like ( P ) d2x = ( P - 2 * P + P ) / hx / hx d2x = ( P - 2 * P + P_left ) / hx / hx d2x = ( P_right - 2 * P + P ) / hx / hx d2y = ( P - 2 * P + P ) / hy / hy d2y = ( P - 2 * P + P_bottom ) / hy / hy d2y = ( P_top - 2 * P + P ) / hy / hy return d2x + d2y + 5 * cosh ( P ). Import numpy as np from scipy.optimize import root from numpy import cosh, zeros_like, mgrid, zeros # parameters nx, ny = 75, 75 hx, hy = 1. This will work just as well in case of univariate optimization: nfev = funcalls, success = ( niter > 1 )) > x0 = > res = minimize ( rosen, x0, method = custmin, options = dict ( stepsize = 0.05 )) > res. return OptimizeResult ( fun = besty, x = bestx, nit = niter. while improved and not stop and niter = maxfev. maxiter = 100, callback = None, ** options ). def custmin ( fun, x0, args = (), maxfev = None, stepsize = 0.1.
